Machine Learning Chapter 7. Computational Learning Theory Tom M. Mitchell. - ppt download
Descrição
3 Computational Learning Theory (2/2) What general laws constrain inductive learning? We seek theory to relate: –Probability of successful learning –Number of training examples –Complexity of hypothesis space –Accuracy to which target concept is approximated –Manner in which training examples presented
(maximum over all possible c C, and all possible training sequences) Definition: Let C be an arbitrary non-empty concept class. The optimal mistake bound for C, denoted Opt(C), is the minimum over all possible learning algorithms A of M A (C)..
(maximum over all possible c C, and all possible training sequences) Definition: Let C be an arbitrary non-empty concept class. The optimal mistake bound for C, denoted Opt(C), is the minimum over all possible learning algorithms A of M A (C)..

Behavior of tropopause height and atmospheric temperature in

SOLUTION: Machine learning tom mitchell - Studypool
ARTIFICIAL INTELLIGENCE AND MACHINE LEARNING (18CS71)

2021 ISHNE/HRS/EHRA/APHRS Expert Collaborative Statement on

Machine Learning : Mitchell, Tom M. (Tom Michael), 1951- author

Functional Traits 2.0: The power of the metabolome for ecology

Machine learning ppt
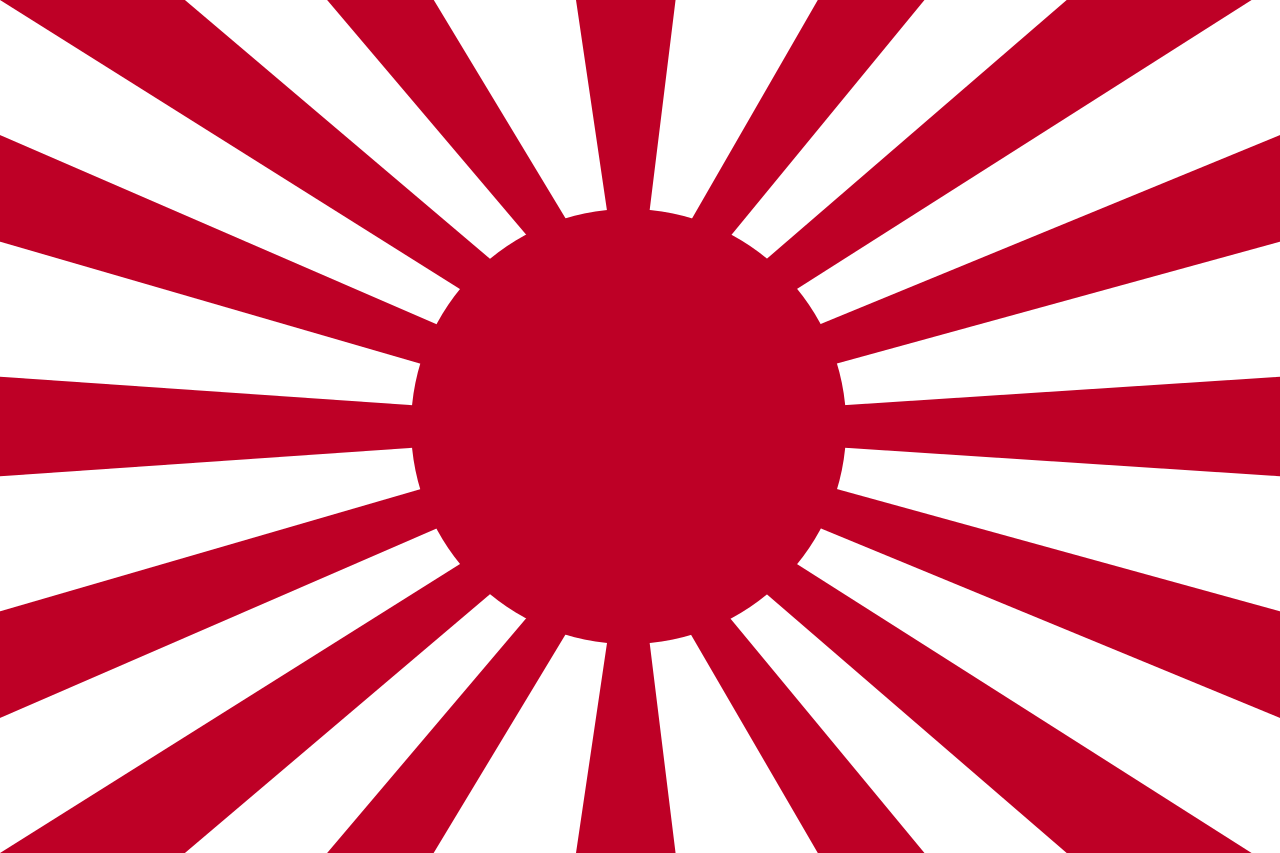
Empire of Japan, Kaiserland Wiki
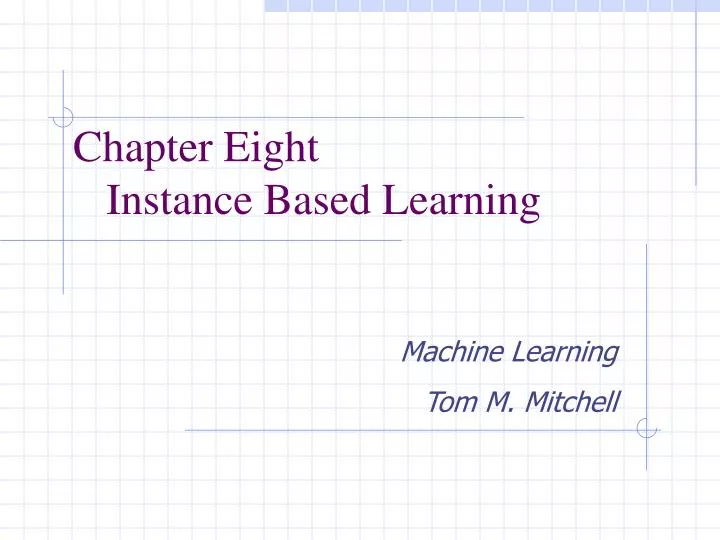
Machine Learning By Tom Mitchell Ppt - Colaboratory
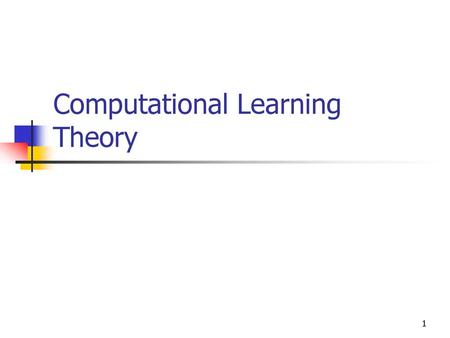
Machine Learning Chapter 7. Computational Learning Theory Tom M
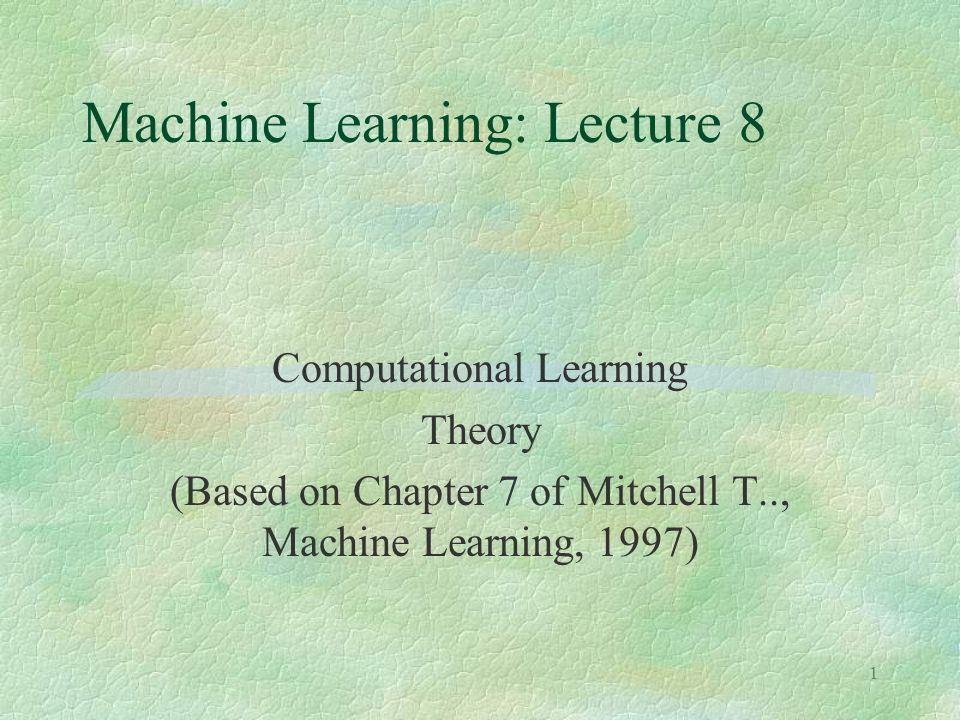
1 Machine Learning: Lecture 8 Computational Learning Theory (Based
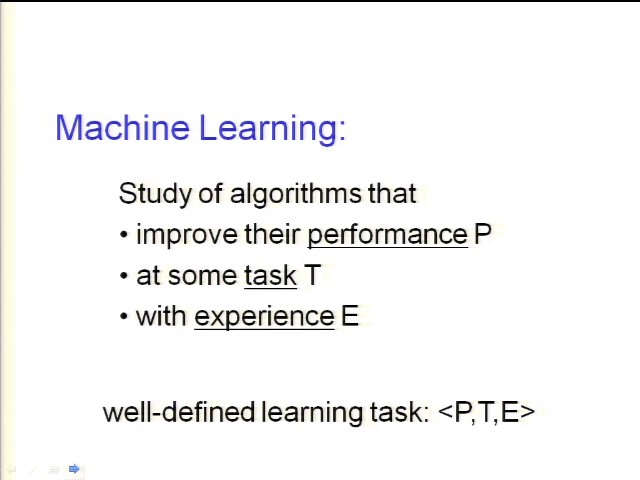
Intro to Machine Learning- Decision Trees By Tom Mitchell

20 Best Artificial Intelligence Books of All Time - BookAuthority
de
por adulto (o preço varia de acordo com o tamanho do grupo)